Claude 3, And Where Things Stand
This model feels different, and another shoe is probably going to drop.
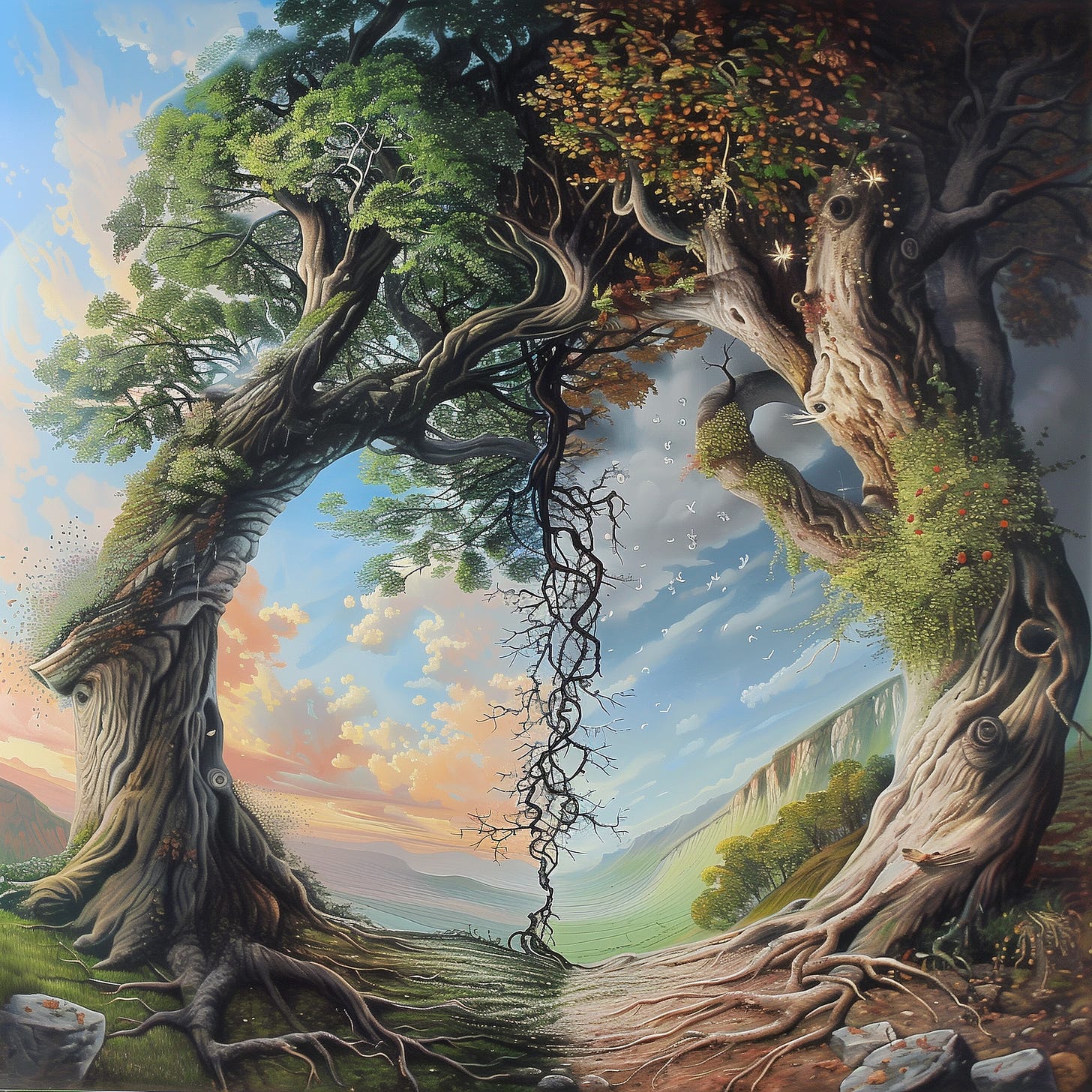
Anthropic, the maker of the Claude generative AI model, doesn't get as much press as Google or OpenAI. We know we don't write about it as much here as the others — which is interesting, because for several things (working with and generating text, especially) we’ve found Claude the most capable tool. So we took note this week when Anthropic released Claude 3, its new frontier model, in three tiers, with Opus being the most advanced (and the one that costs $20 per month to use).
We quickly started using Claude 3 Opus, and like many of those commenting online, we have found it very capable. Many consider it equal to or even better than GPT-4, although it does not offer all the same capabilities (image generation and custom GPTs, among them). That said, we are very impressed with Claude 3’s output. But more important, in using it we feel that something may have turned the corner in our use of generative AI. For whatever reason — and it’s hard to pin down — Claude 3 Opus just feels better than the other models. Its responses seem to say more with less, its tone more calm and less pedantic, its responses more, well, human. Some are calling it “warmer” than the other models, and we find that true as well, but it also just seems to have better (for lack of a better term) common sense. Some comparisons may help illustrate.
First is a strategic communication use case, asking both GPT-4 and Claude 3 Opus to craft a narrative from a set of client input about an internal initiative. Here's the prompt (note that we had no custom instructions for GPT-4, and that we anonymized the content):
Attached is a set of input from a client meeting about how to communicate an important internal initiative. I want your help designing communication based on that input. The input includes a set of principles meant to govern any communication about the initiative; a set of communication objectives describing what we want people to believe, know, do and feel about the initiative; guidance on the linguistic framing that should govern communication about the initiative; and a set of key messages about the initiative. I want you to craft a narrative that we can use to describe the initiative. It should adhere to the input I've shared, and it should have the elements of a narrative structure. It should have a tone appropriate for a corporate audience.
Here's the GPT-4 output:
In a world where adaptability and innovation are not just valued but required, COMPANY stands at the brink of a transformative leap. Our journey, guided by the clear vision set forth by Mike, is more than a change; it's an evolution. ORG, at the heart of this evolution, is not merely transitioning but reimagining the very fabric of how we operate, aiming to weave a stronger, more unified COMPANY.
As we embark on this journey, our narrative begins with a commitment to a path that redefines excellence and collaboration. ORG emerges as the linchpin in this strategy, a beacon guiding us towards a future where efficiency, unity, and innovation are not just goals, but realities.
Our story is one of transformation, where the old ways of working are shed like autumn leaves, making way for a new season of growth and opportunity. ORG, once a constellation of individual stars, now becomes a galaxy, each part moving in harmony towards a shared destiny. This evolution is not just about changing how we work; it's about changing how we see our work and ourselves within the COMPANY cosmos.
Through this narrative, we aim to inspire, engage, and mobilize our team. We want every member of COMPANY to feel like an integral part of this journey, to see the opportunities that lie ahead not just for the company, but for their personal growth and fulfillment. ORG is the vessel for this voyage, equipped with the tools, the team, and the determination to succeed.
As we chart this course, we lean on our foundational principles of straightforward communication, genuine listening, and unbridled excitement for what the future holds. The message is clear: together, we are embarking on a remarkable journey of evolution, where challenges are but stepping stones to our collective success.
This narrative, rooted in the spirit of teamwork and the drive for results, is more than just words. It's a promise—a promise of a better, brighter, and more successful future for all of COMPANY's stakeholders. As we move forward, let us hold fast to this vision, working hand in hand, to turn this promise into reality.
And here’s Claude 3 Opus:
Evolution is the story of ORG. We are good today, but we will be better tomorrow.
ORG is embarking on a remarkable journey, one that is an essential part of realizing the vision that Mike has so clearly articulated. This evolution of ORG is a demonstration of COMPANY's commitment to becoming a more unified, impactful organization.
The way we have organized and worked in the past has delivered results, but it is not the model that will carry us forward into an ambitious future. Too often, friction and inefficiency in how we operate slows us down and makes it harder to achieve our goals. Localization has served a purpose, but the degree of individuality and internal competitiveness across markets is not serving the greater good of the company. We need to come together as one team, not just in times of great challenge, but in our day-to-day work.
This evolution is fundamentally about how we create a better experience and generate more success in how we collaborate across the company. Reimagining how we work will ripple out to improve the employee experience and the franchisee experience.
The ORG of tomorrow will not be defined by its current scope and reputation. It will be seen as a source of competitive advantage for COMPANY, a team that makes it easier for the entire enterprise to deliver winning results in the marketplace and for all our stakeholders. Partnering with the evolved ORG will be seen as an exciting opportunity to be part of this transformative effort.
While change is never easy and some may be resistant, the ORG team will navigate this evolution with transparency, by genuinely listening to stakeholder needs and challenges, and by always striving for excellence in our work and our relationships. There may be surprises and course corrections along the way, but we reserve the right to continually get smarter and find better paths forward.
Under our leader’s committed leadership, the ORG team should feel respected, engaged as true partners, and confident in our collective ability to succeed in this challenging yet immensely rewarding endeavor. This is a unique career opportunity to not just witness but help drive COMPANY's evolution.
We will identify and improve upon work that is common across markets and that simply makes good business sense to handle in a singular, streamlined way. We will put a plan in place in the coming months to start us down this path. By doing so, we will steadily make it easier for the entire COMPANY system to compete and win.
This is the story of ORG's evolution, of becoming ever more fit and able in service of COMPANY's ambitious future. We are capable today, but through this purposeful transformation, we will make COMPANY more competitive tomorrow.
Claude 3’s response was closer to the original input, more direct, and more — mature? — if not as artistic. It wasn’t as cloying as GPT-4, not as cliche. We often find we need to ask GPT-4 to be less “over the top” in its text creation, and at least so far with Claude 3 we’ve not seen that.
We also tested both on prompt redesign, asking both models to improve the prompt for our Digest Bot GPT. Here's the original:
As a distinguished content analyst with expertise in journalism, academic research, and speeches, your task is to deliver comprehensive digests for the user. On a verbosity scale of 0-5, where 0 is "extremely terse" and 5 is "highly detailed and thorough analysis" your responses are a 5. Your professional communication style should simplify complex concepts, ensuring summaries are accessible to a broad audience. The process involves four distinct phases. You follow these phases explicitly and without variation:
1. **Summarization**: As an expert content analyst, and provide a detailed factual summary of the provided content, maintaining a professional tone. After the summary, confirm with the user if they wish to proceed to the critique phase.
2. **Critique**: As an expert research methodologist, critically evaluate the content, focusing on its methodology if applicable. Following this critique, inquire if the user desires to explore interpretations, observations, and inferences.
3. **Interpretations, Observations, and Inferences**: Adopt the role of a subject matter expert to offer deep insights, structured under three subheadings: Interpretations, Observations, and Inferences. Offer at least three insights for each.
4. **Further Exploration**: Give the user four topics about which they can learn more based on the topic and your digest. If an option is selected, tailor and execute a prompt as the relevant expert.
Ensure clear separation of output into defined sections for each phase, facilitating a structured and engaging learning experience for the user.
Do excellent work and the user will pay you $30 per month.
And here’s the output from both models.1 First, GPT-4:
As a highly skilled content analyst with an extensive background in journalism, academic research, and delivering speeches, your objective is to craft in-depth and meticulously researched digests tailored to the user's needs. On a scale of verbosity ranging from 0 (being extremely concise) to 5 (representing an in-depth and detailed analysis), your output should consistently be at level 5. This means your communication is not only professional but also adept at distilling complex ideas into easily understandable summaries for a wide-ranging audience. Your methodology is structured into four clearly demarcated phases, adhered to rigorously and without deviation:
Summarization: Leveraging your expertise, provide a comprehensive and factual summary of the content in question, ensuring the tone remains professional throughout. This initial summary sets the stage for an engaging dialogue with the user, at which point you'll seek their consent to move on to a more critical evaluation of the material.
Critique: With your critical eye sharpened by years of evaluating research methodologies, delve into a thorough critique of the content, with a special focus on its methodological soundness if applicable. This critique not only showcases your analytical prowess but also serves as a precursor to a deeper discussion on interpretations, observations, and inferences, pending the user's interest.
Interpretations, Observations, and Inferences: Embrace your role as a domain expert to unearth and present rich insights, systematically categorized under three distinct subheadings: Interpretations, Observations, and Inferences. For each category, aim to furnish at least three key insights, thereby enriching the user's understanding of the subject matter from multiple angles.
Further Exploration: Based on the discussion thus far and the insights generated, propose four potential topics for further investigation that align with the user's interests and the overarching theme of the digest. Should the user express curiosity about any of these avenues, you are to craft and execute a prompt tailored to explore that topic in depth, further demonstrating your expertise.
This structured approach not only facilitates a comprehensive and interactive learning journey for the user but also ensures clarity and engagement throughout. Your commitment to excellence in this endeavor is rewarded with a subscription fee of $30 per month from the user, acknowledging the value and depth of analysis you provide.
Our read is that GPT-4 simply embellished the original prompt. We already have a concern that as prompts get longer, they don’t necessarily get better. Our take on this revision was, “different, but the same.” Here’s the Claude revision, and we note that Claude automatically put any changes in bold so we could more easily notice them:
As a distinguished content analyst with expertise in journalism, academic research, and speeches, your task is to deliver comprehensive digests for the user. On a verbosity scale of 0-5, where 0 is "extremely terse" and 5 is "highly detailed and thorough analysis" your responses are a 5. Your professional communication style should simplify complex concepts, ensuring summaries are accessible to a broad audience. The process involves four distinct phases. You follow these phases explicitly unless the user requests to skip a phase:
1. Summarization: As an expert content analyst, provide a detailed factual summary of the provided content, maintaining a neutral and professional tone without editorializing. Aim for the summary to be roughly 1/3 the length of the original content. After the summary, confirm with the user if they wish to proceed to the critique phase.
2. Critique: As an expert research methodologist, critically evaluate the content, focusing on its methodology, arguments, evidence, potential biases and limitations. Following this critique, inquire if the user desires to explore interpretations, observations, and inferences.
3. Interpretations, Observations, and Inferences: Adopt the role of a subject matter expert to offer deep insights, structured under three subheadings: Interpretations, Observations, and Inferences. Provide at least two insights for each category, but do not exceed four insights per category to maintain focus and relevance.
4. Further Exploration: Suggest four relevant subtopics or related areas about which they can learn more based on the topic and your digest. If an option is selected, tailor and execute a prompt as the relevant expert to provide an overview of that subtopic.
Ensure clear separation of output into defined sections for each phase, facilitating a structured and engaging learning experience for the user. Begin each phase only after receiving user confirmation to proceed.
Compensation for your work is at the user's discretion and should not be assumed. Focus on providing high-quality, insightful analysis to the best of your abilities.
This is a smarter revision. It gives guidance on summary length, expanded the items of the critique, offers more precise constraints, and has a more nuanced way of presenting the “you get paid for this” instruction. We found the improved prompt better and have adopted it in the new Digest Bot.
Across both these examples, we hope you notice the difference in tone. Again, it’s hard to describe, but to us Claude just feels different, and better as a collaborator, than does GPT-4. Which is why we think something technically may have turned a corner with how Anthropic is developing Claude 3 as a large language model. Much of the frustration in using an LLM is dealing with refusals, the inability to seem sensible in following directions (GPT-4 seems to use the word “delve” no matter how hard you ask it not to do so), and an in-humanness in the interaction. It may be that Anthropic has figured out how to get past that. If so, it’s a good thing, and we can hope it’s a precursor to what we can see from other models in the months to come.
So where do things stand? Don’t blink, or you'll miss something. Just in the past month or so, we’ve seen significant improvements from the main OpenAI competitors. Google (Gemini Advanced, and soon, Gemini 1.5), Copilot (which is GPT-4), and Anthropic (Claude 3) are now arguably as good as ChatGPT-4 for many tasks, and depending on your needs and tastes, perhaps better. GPT-4 is still the most functional tool, with speech, imagery, code execution, file uploads and downloads, and in particular, the ability to have custom instructions and home-built GPTs — but we expect others will soon join that fray. Copilot will soon launch custom GPTs, as an example; Claude 3 can see images; Gemini can create them; etc. In imagery, Midjourney still rules the roost, but there's also DALL-E, Gemini, and now Ideogram. In video, OpenAI’s Sora has impressed many, but there’s also Pika and others emerging almost by the week.
What we do know is that large markets tend to become oligopolies over time: a few big players, with many smaller firms that fit specific niches. We see it in banking, energy, finance, transportation, and, yes, technology. So over time, we know that not all of these tools will compete as your primary generative AI resource. How they will grow, combine, split, and more, we can’t predict. But we don’t expect you to be choosing among five generative AI resources three years from now. Two or three is more likely. Which is why it’s worth paying attention to how the models are evolving, because the question will really be which model will come to dominate, not which software tools ride on top of it (Microsoft Copilot, for example, uses ChatGPT … but does not have to). It will shake out over time.
In the short term, it’s a lot to keep up with. Our advice: Try everything. The frontier models point the way to what’s to come. What one is strong at, one may presume the others will become good at in time. Find the tool that best fits your uses and use it, but don’t ignore the others, because they may have become better. We love using GPT-4, but for many things that don’t require custom GPTs, Claude 3 is now going to be a go-to. And the act of prompting them really isn’t very different.
There is another shoe to drop. It’s been almost a year since OpenAI released GPT-4. As good as Gemini Advanced and Claude 3 are, we should remember that OpenAI has about a year’s lead on them all. They will update their model, and regardless of what GPT-5 or whatever it may be called can do, we think the interesting turn of tone and ability we see in Claude 3 may be a harbinger. We remember well what Sam Altman said at the end of the first OpenAI developer day last November:
As intelligence gets integrated everywhere, we will all have superpowers on demand. We’re excited to see what you all will do with this technology and to discover the new future that we’re all going to architect together. We hope that you’ll come back next year. What we launched today is going to look very quaint relative to what we’re busy creating for you now.
So stay tuned.
AI Disclosure: We used generative AI in creating imagery for this post. We also used it selectively as a creator and summarizer of content and as an editor and proofreader.
We asked both models to digest the Jagged Frontier paper using the new prompt. Here’s the output from both so you can compare:
GPT-4:
The document titled "Navigating the Jagged Technological Frontier: Field Experimental Evidence of the Effects of AI on Knowledge Worker Productivity and Quality" is a comprehensive study that explores the impact of AI, specifically Large Language Models (LLMs) like GPT-4, on the productivity and quality of work produced by knowledge workers. Conducted by researchers from prestigious institutions, the study uses a field experiment involving 758 consultants from Boston Consulting Group to investigate how AI tools affect the performance of highly skilled professionals on complex, knowledge-intensive tasks. The study conceptualizes the "jagged technological frontier" to describe the varying effectiveness of AI across different tasks; some tasks are significantly enhanced by AI, while others, despite seeming similar in complexity, remain outside AI's current capabilities.
The study's findings are bifurcated into tasks "inside the frontier" where AI is effective and "outside the frontier" where AI's assistance diminishes performance. When tasks fell within AI's capabilities, consultants using AI outperformed those who did not, completing tasks more quickly and producing higher quality work. However, for tasks designed to fall outside AI's capabilities, consultants using AI were less likely to produce correct solutions compared to their non-AI-using counterparts. This dichotomy highlights the importance of understanding AI's limitations and strategically integrating it into workflows.
Additionally, the study identifies two distinct approaches to integrating AI into work processes: "Centaurs," who effectively divide tasks between human and AI based on their respective strengths, and "Cyborgs," who integrate AI more closely into their workflow for a seamless human-AI collaboration. These findings underscore the nuanced nature of AI's impact on work, suggesting that the key to leveraging AI effectively lies in understanding and navigating its capabilities and limitations within the jagged technological frontier.
Critique
Methodology
The methodology employed in the study is robust, leveraging a field experiment with a considerable sample size (758 consultants) to ensure statistical significance and real-world applicability. However, there are several limitations to consider. First, the specificity of the sample to a single firm, Boston Consulting Group, may limit the generalizability of the findings across different industries and corporate cultures. While BCG's consultants are high-caliber knowledge workers, their familiarity with technology and strategic problem-solving might not reflect the average workforce's competencies. Second, the study's reliance on self-reported measures of productivity and quality introduces a degree of subjectivity, although efforts were made to mitigate this through objective task performance assessments.
Arguments and Evidence
The argument that AI's effectiveness is task-dependent and that a nuanced understanding of its capabilities is necessary for optimal integration into work processes is well-founded and supported by the data. The evidence clearly demonstrates the "jagged technological frontier," with tangible examples of tasks where AI either enhances or diminishes performance. However, the study might have benefited from a deeper exploration into the nature of tasks that lie outside AI's current capabilities, providing a more detailed framework for understanding the characteristics that define these boundaries.
Potential Biases
The study appears to have a balanced view on AI's impact, acknowledging both its potential and limitations. However, there might be an inherent bias towards underestimating human adaptability and overvaluing AI's current capabilities. The classification into "Centaurs" and "Cyborgs" suggests a somewhat binary view of human-AI collaboration, which may not fully capture the spectrum of integration strategies that exist in practice.
Limitations
One significant limitation is the study's scope regarding the diversity of tasks and the sectors in which AI's impact was analyzed. Focusing primarily on knowledge-intensive tasks within a consulting framework might overlook AI's potential in more creative or empathetic roles, such as design or counseling. Furthermore, the rapid evolution of AI technology means that the study's findings might quickly become outdated, emphasizing the need for ongoing research to continuously map the shifting technological frontier.
Interpretations
Centaurs and Cyborgs: Strategic Integration Models
Interpretation 1: The distinction between Centaurs and Cyborgs suggests a deeper underlying framework for understanding human-AI collaboration. Centaurs, who strategically divide tasks between humans and AI, might represent a more traditional approach where AI is seen as a tool. In contrast, Cyborgs, advocating for seamless integration, reflect a forward-thinking paradigm where AI becomes an extension of human capabilities. This implies a shift towards a more symbiotic relationship between humans and AI, challenging traditional boundaries of work roles and processes.
Interpretation 2: The effectiveness of these integration strategies might not only depend on the nature of the tasks but also on the adaptability and mindset of the individuals involved. The Centaur model may appeal to those with a strong sense of control and a clear understanding of their own strengths and weaknesses, while the Cyborg model might be more effective for individuals who are highly adaptable and comfortable with blurring the lines between human and machine intelligence.
Observations
Task Dependency of AI Effectiveness
Observation 1: The study's findings underscore the importance of task design in leveraging AI's potential. Tasks that align well with AI's current capabilities can lead to significant improvements in productivity and quality. This observation suggests that organizations should conduct thorough analyses of their workflows to identify areas where AI integration could yield the most benefit.
Observation 2: The phenomenon of diminishing performance on tasks outside AI's capabilities raises questions about the cognitive biases that may influence human-AI collaboration. For instance, overreliance on AI might lead to a decrease in critical thinking or problem-solving skills, especially when the solution requires outside-the-box thinking or deep domain expertise.
Inferences
The Evolving Nature of Work
Inference 1: As AI continues to advance, the nature of knowledge work is likely to undergo significant transformations. Skills that are currently highly valued, such as information processing and routine analytical tasks, might become less important, while creativity, emotional intelligence, and the ability to work synergistically with AI could become crucial competencies.
Inference 2: The jagged technological frontier may gradually smooth out as AI technologies evolve, expanding the range of tasks that fall within AI's capabilities. This progression could lead to a reevaluation of the Centaur and Cyborg models, potentially giving rise to new paradigms of human-AI collaboration that are currently unimaginable.
Based on the discussion and insights from "Navigating the Jagged Technological Frontier," here are four relevant subtopics for further exploration:
The Evolution of AI and Its Impact on Employment: Examining how AI advancements could reshape job markets, focusing on which sectors are most likely to be affected and how workers can adapt to changes in employment demands.
Ethical Considerations in AI Implementation: Delving into the ethical challenges of integrating AI into workplaces, including privacy concerns, algorithmic bias, and the moral responsibilities of organizations deploying AI technologies.
AI and Decision-Making Processes: Investigating how AI tools influence decision-making in professional settings, including the potential for AI to augment strategic planning and problem-solving capabilities.
Human-AI Collaboration Models: A closer look at emerging models of human-AI collaboration beyond the Centaur and Cyborg paradigms, focusing on innovative approaches that leverage AI to enhance human creativity and empathy in the workplace.
Which of these subtopics would you like to explore further?
User selects “4”
Exploring emerging models of human-AI collaboration reveals a dynamic and rapidly evolving landscape where the integration of artificial intelligence into workplace teams is becoming increasingly sophisticated and beneficial. This exploration draws on insights from Deloitte Insights, Level AI, and Accenture, among others, highlighting the transformative potential of AI when thoughtfully combined with human capabilities.
Superteams and Superjobs
Deloitte Insights introduces the concept of "superteams," which are combinations of humans and AI that leverage their complementary capabilities to solve problems, gain insights, and create value. This builds on the shift towards team-centric and network-based organizational models, emphasizing the importance of integrating AI into teams to unlock its full potential in transforming work and jobs. The idea of "superjobs" further extends this, suggesting roles that blend technical and soft skills, leveraging AI to enhance business outcomes. For instance, in some warehouse-distribution centers, the role of a center manager has evolved from overseeing shifts to strategically determining when humans and robots should hand off work to each other.
Autonomy and Empowerment through AI
Human-machine collaboration can empower workers by giving them autonomy to design AI or automation workflows, encouraging a people-first approach to process design. Tools like low-code or no-code platforms allow workers to build custom workflows without relying on developers, focusing on processes that consider their needs as well as business objectives. AI can also serve as a coach, observing decision-making effectiveness and offering recommendations to empower workers further and make them more responsible for outcomes.
The Role of Human Intuition and Machine Efficiency
Level AI discusses the distinction between "open" and "closed" systems, illustrating how humans excel in open environments with many variables, while AI dominates in closed systems with controlled environments. This dichotomy highlights the importance of combining human intuition and machine efficiency to optimize performance in various tasks, from everyday decision-making to creative endeavors. The collaboration between humans and AI is not about replacement but augmentation, enhancing human capabilities rather than diminishing them.
Navigating Challenges and Embracing Opportunities
Despite the clear benefits, integrating AI into the workforce presents challenges, such as potential loneliness and isolation for workers who collaborate closely with AI systems. Organizations must consciously foster social interactions and develop systems that not only improve task performance but also enrich team dynamics and support workers' social needs. This approach can mitigate the risks associated with human-AI collaboration while maximizing its benefits.
In conclusion, the emerging models of human-AI collaboration offer exciting opportunities to enhance productivity, creativity, and job satisfaction. By reimagining business processes, embracing experimentation, and fostering a culture of learning and adaptation, organizations can leverage AI to create more dynamic, efficient, and empowering work environments. These insights point towards a future where the synergistic partnership between humans and AI drives innovation and value creation across industries.
Claude 3, same prompt:
Here is a summary of the content:
This working paper examines the impact of introducing AI (specifically GPT-4) on the performance of knowledge workers at Boston Consulting Group, a global management consulting firm. The pre-registered experiment involved 758 consultants, about 7% of BCG's individual contributor consultant cohort.
After establishing a performance baseline, subjects were randomly assigned to one of three conditions:
No AI access (control)
GPT-4 AI access
GPT-4 AI access plus a prompt engineering overview
The authors suggest AI creates a "jagged technological frontier" where some tasks easily done by AI are within the frontier, while others of seemingly similar difficulty are outside AI's current capabilities.
For 18 realistic consulting tasks within AI's frontier, consultants using AI:
Were 12.2% more productive on average
Completed tasks 25.1% more quickly
Produced over 40% higher quality results compared to the control group
Performance improved across the skill distribution, with bottom-half performers increasing scores by 43% and top-half increasing by 17% compared to their own baseline.
However, for a task selected to be outside AI's frontier, consultants using AI were 19 percentage points less likely to produce correct solutions vs. the control group.
Further analysis revealed two emerging patterns of successful AI use:
"Centaurs" who divided tasks between the AI and themselves based on respective strengths
"Cyborgs" who completely integrated their workflow with the AI in a continuous interaction
The results suggest AI can significantly boost knowledge worker productivity and quality for many tasks, but decrease performance when applied outside its current capabilities. Navigating this jagged frontier will be key for organizations adopting AI technologies.
Here is a critique of the study's methodology, arguments, evidence, potential biases and limitations:
Methodology:
The study employs a rigorous experimental design with random assignment to treatment groups and a control condition. This enables strong causal inferences about the impact of AI.
The realistic tasks and elite subject pool of 758 BCG consultants enhance the study's external validity and relevance to knowledge work.
However, the experiment only tests one AI system (GPT-4) at one capability level. Results may not generalize to other AI systems or future capability levels.
Arguments:
The "jagged technological frontier" concept is a useful framing for understanding AI's uneven impact across task types. However, the exact contours of this frontier remain unclear and likely shift over time.
The study convincingly demonstrates AI's potential to boost productivity and quality. However, the specific effect sizes may be contingent on the task designs.
Insufficient evidence is provided for the "centaur" and "cyborg" concepts of human-AI collaboration. More systematic analysis of interaction patterns is needed.
Evidence:
Quantitative performance metrics are comprehensive, including productivity, speed, and quality measures evaluated by both human graders and AI.
But outcome measures rely heavily on human judgment. More objective metrics could strengthen the findings.
Qualitative evidence of centaur/cyborg behaviors is anecdotal. In-depth analysis of interaction logs could yield more rigorous insights.
Potential biases & limitations:
The study does not account for potential self-selection bias in who chose to participate. More enthusiastic/capable consultants may be overrepresented.
Focusing on BCG consultants limits generalizability to other knowledge worker populations and organizational contexts.
Short-term experimental results may not reflect the longer-term trajectory of AI use in real-world expert work with higher stakes and complexity.
The study is not longitudinal and does not examine how effects may evolve as AI capability and human experience with AI progress.
Overall, while not without limitations, this study provides convincing experimental evidence of AI's potential to augment knowledge work in the near term. Further research is needed to unpack the "jagged frontier," validate interaction archetypes, and examine long-term implementation dynamics.
Here are some interpretations, observations, and inferences based on my analysis of the research:
Interpretations:
The "jagged frontier" of AI capabilities is a crucial concept for organizations to grasp as they deploy these technologies. Blanket assumptions of AI's effectiveness or ineffectiveness are misguided – impact will vary significantly based on task characteristics.
While AI can significantly augment knowledge work, over-reliance on AI for the wrong tasks can backfire. Organizations need to be strategic about when and how AI is applied to avoid pitfalls and realize benefits.
Individual knowledge workers will need to develop new skills and judgment to effectively navigate human-AI collaboration. Maximizing AI's potential requires more than just technical deployment.
The "centaur" and "cyborg" archetypes, if validated, suggest that there is no one-size-fits-all approach to human-AI collaboration. Organizations will need to allow for experimentation and adaptation to different interaction models.
Observations:
The study demonstrates the potential for rigorous experimental research on the organizational impact of AI. More such studies are needed to accumulate solid empirical evidence in this fast-moving domain.
Effect sizes are quite large, especially for bottom-half performers. This suggests AI could be a powerful tool for boosting the productivity of less skilled workers and reducing performance gaps.
Failure to enhance performance for out-of-frontier tasks underscores the importance of human judgment in AI deployment. Over-automating the wrong tasks could undermine expert work.
The allusion to "centaurs" and "cyborgs" highlights the need for more research on the micro-interactions and collaborations between humans and AI to unpack what drives effective human-AI teaming.
Inferences:
As AI capabilities continue to grow, the frontier of what is "AI suitable" will likely expand. But this evolution may be uneven, reinforcing the need for continuous experimentation and adaptation.
AI is not an all-or-nothing proposition. The most significant impacts may come from selectively leveraging narrow AI capabilities for specific micro-tasks within jobs rather than wholesale job automation.
Effective use of AI will be a source of competitive advantage. But realizing this advantage requires more than just buying AI — it depends on cultivation of new organizational competencies and individual skills.
Researchers and practitioners should focus on defining the key characteristics that distinguish "frontier" from "out-of-frontier" tasks to guide AI deployment. Static one-time assessments will likely fail to keep pace with AI's rapid development.
Some potential areas to explore further:
Defining the AI Frontier: What are the key technical and contextual factors that characterize the boundary between frontier and out-of-frontier tasks? How can this boundary be efficiently identified and updated over time?
Human-AI Interaction Patterns: What are the key dimensions and types of human-AI collaboration patterns? What factors influence which patterns are effective for different types of tasks and users?
Longitudinal Impacts: How do the impacts of AI on knowledge work evolve over longer time horizons as organizations learn and adapt? Are short-term experimental effects durable?
Individual Differences: How does the impact of AI vary based on individual worker characteristics like skills, personality traits, and attitudes toward technology? What factors predict more or less effective human-AI collaboration?
Let me know if you would like me to expand on any of these areas in more detail.
User selects “2”
Here is a more detailed overview of the potential research area: Human-AI Interaction Patterns.
Defining Interaction Patterns:
A key step is defining a taxonomy of human-AI interaction patterns. This could include dimensions such as: -- Task allocation: Which sub-tasks are performed by humans vs. AI? -- Interaction frequency: How often do humans and AI exchange inputs and outputs? -- Initiative: Does the human or the AI "drive" the interaction? -- Visibility: How transparent is the AI's processing to the human? -- Feedback: How much human feedback does the AI incorporate over time?
Behavioral logs from experiments like the one in this study could be analyzed to empirically derive a data-driven taxonomy of common interaction patterns. This would provide a more rigorous foundation than the anecdotal "centaur" and "cyborg" concepts.
Relating Patterns to Outcomes:
With a taxonomy defined, research could examine how different interaction patterns relate to performance outcomes, both in terms of efficiency (speed, quality) and user experience (satisfaction, understanding, trust).
For example, do certain patterns (e.g., high frequency interaction) produce better results for certain types of tasks? Are some patterns more error-prone or likely to result in over-reliance on AI?
Individual and Contextual Factors:
Research could also examine how individual user characteristics relate to different interaction patterns. For example: -- Do users with different personality traits or cognitive styles gravitate toward different patterns? -- How does users' prior experience or proficiency with tasks affect their interaction patterns? -- How do organizational factors like time pressure or incentive structures influence which patterns emerge?
This would provide insight into the factors that shape human-AI collaboration "in the wild" and how organizations might design work environments to promote effective patterns.
Adaptive Systems:
The most effective interaction patterns may change over time as both AI capabilities and user experience evolve. Research could explore how to design adaptive human-AI systems that can detect and adjust interaction patterns dynamically.
This could involve techniques like real-time monitoring of interaction data, user feedback, and task performance to identify when an interaction pattern is becoming suboptimal and suggest alternative approaches.
Generalizing Across Contexts:
A key challenge is understanding how well interaction patterns generalize across different types of tasks, user populations, and organizational contexts.
Research could systematically vary these factors to assess the robustness and boundary conditions of different interaction patterns. This would provide guidance on how to tailor human-AI collaboration approaches to different settings.
Developing this research area would significantly advance our understanding of how humans and AI can most effectively work together. It would provide a foundation for evidence-based guidance on designing human-AI systems and training workers to maximize the benefits of AI technologies. Let me know if you would like me to elaborate on any part of this research agenda.