Confluence for 10.8.2023
Two days with Wharton’s Dan Rock. Bing Chat Enterprise is rolling out. New paper on GPT-4 with vision — you need to see it to believe it.
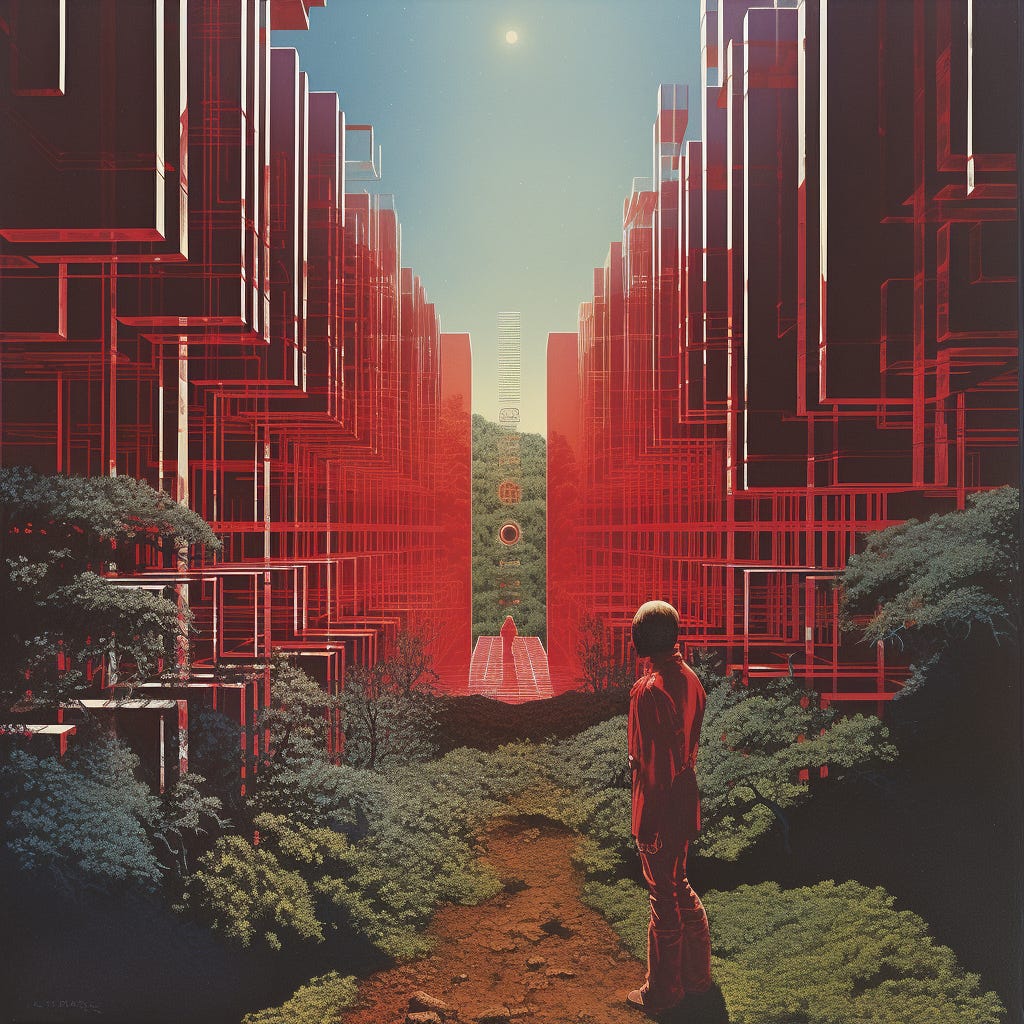
Welcome back to Confluence. Several of us are just back from a week-long retreat that we host for senior clients every year in northern Wisconsin. AI was an area of focus this year, and we are full of insights and ideas that we hope to present here over the coming weeks. In the meantime, here’s what has our attention at the intersection of generative AI and corporate communication — and because we have some longer pieces in this issue, we have three updates rather than five:
Two Days with Wharton’s Dan Rock
Bing Chat Enterprise Is Rolling Out
New Paper on GPT-4 with Vision — You Need to See It to Believe It
Two Days with Wharton’s Dan Rock
We learned much from the economist about what generative AI may mean for corporate communication and organizational life in general.
As noted above, we’ve just hosted our annual client retreat, and our agenda included two days with Wharton economist Dan Rock. Dan is among the leading thinkers on the economic implications of automation, and we’ve posted about his research on the labor impacts of generative AI in the past.
We hope to interview Dan over the next few months and share some of his thinking in a special issue. Until then, here are seven notions we took away from our time with him:
Generative AI qualifies as a general purpose technology. In economics these are technologies that have pervasive impact, improve over time, and both require and spawn complementary innovation. They have an outsized impact in the research and development portion of the economy, which creates extensive downstream ripple effects across the economy and technology more broadly. We have not had many such technologies in human history, but the steam engine, electricity, and the transistor are among them.1
With these technologies, eventually the cost of what they make more efficient gets very low, and can even go to zero, while costs in the areas of the economy that they can’t make more efficient expand.2 Example: With everyone able to self-publish via social media there is an explosion of content and it’s cheap or free to get … so the focus shifts from creating content (easy and cheap) to commanding attention (hard). It is a useful thought experiment to extend this thinking to corporate communication, which we intend to do.
People are misinterpreting what it means when the research says there is “overlap” or “exposure” of specific tasks and jobs to generative AI. In Dan’s research “exposure” means, “Could I double my productivity on this task without a notable drop in quality?” For many tasks in corporate communication “exposure” actually means the technology should augment what one is able to do, perhaps significantly, not replace it (although there are tasks where replacement occurs).
It is difficult for generative AI to present exposure where the person has information that is not in the data on which the model was trained. In the case of GPT-4, for example, if you know things that are not on the Internet (which is the basis of its training), or not widely found on the Internet, then you have content the model can’t easily produce or replace. Knowing things of value that other people don’t know has always been a source of power, and it is with large language models as well.
Us: “Dan, how do you think about using GPT-4?” Dan: “I think of it as having hordes of interns.” He uses it to organize, summarize, read and review content, critique thinking, and come up with ideas. He does not use it to create content that has reputational consequences, to do citations, to do complex work, or tasks that require distinctive expertise.
We have a conclusion we drew from talking with Dan, which is not his own, but is worth noting: from what he described we can expect tools like GPT-4 to create a dispersion of some tasks currently “owned” by corporate communication out into the larger organization. Content creation is an obvious example. If everyone in the company can now create pretty good, or even really good, content thanks to tools like GPT-4, Midjourney (and soon, videos and music through similar technology), the role of corporate communication will need to change in some capacity from being the creators of content to being the shepherds of quality, brand standards, and governance.
We should be prepared for a long period of change, but should also have a grain of humility — with general purpose technologies there is much we can’t predict, and people who say they have it all figured out, probably don’t.
Bing Chat Enterprise Is Rolling Out
Employees may have access to generative AI within your corporate firewall and security architecture sooner than you think.
We forecasted last week that Copilot and GPT-4-powered generative AI capabilities were set to arrive from Microsoft in November. That said, users in our Office365 instance seem to already have secure AI chat via Bing (which we can access via the Edge browser or by using Bing chat at www.bing.com). This means the tool is within our Microsoft-sourced IT infrastructure, keeps our data private and secure, runs off GPT-4, and includes image creation ability with DALL-E. We are putting it through its paces but so far, we are impressed, and it solves many of our security and privacy concerns.
We do wish it supported custom prompts and allowed aspect ratios other than 1:1 for image creation. That said, so far the quality of responses is impressive and as we continue to use Bing and OpenAI side-by-side, we’ll share our impressions here. For those in corporate communication, this also means, though, that many of your employees may soon have access to generative AI capabilities even without having access to OpenAI’s version of GPT-4. We recommend consulting your IT department to learn whether this capability is on their near-term roadmap (or is perhaps already live).
New Paper on GPT-4 with Vision — You Need to See It to Believe It
OpenAI’s generative AI tool is about to be as capable with images as it is with text.
Last week, we wrote a bit about the “multi-modal” turn — the evolution in generative AI from working only with text to working with both text and images (and eventually, sounds and even smells). It will soon understand, reason with, and offer answers or responses about images just as it does with text. This includes solving problems (example: given a mis-ordered set of photos of people making sushi, GPT-4V placed them in the correct order; another: shown a photo of a leaky faucet, GPT-4V provided the correct fix; another, more impressive: given a set of x-rays, GPT4-V made the correct radiological interpretation). The paper is long, so here’s the Bing Enterprise (with GPT-4) summary:
The paper is titled “The Dawn of LMMs: Preliminary Explorations with GPT-4V(ision)” and it was published on September 29, 2023 by researchers from Microsoft and OpenAI. The paper analyzes the latest large multimodal model (LMM), GPT-4V, which extends the large language model (LLM), GPT-4, with visual understanding capabilities. The paper presents a collection of test samples that demonstrate the intriguing tasks that GPT-4V can perform, such as:
Solving math problems that involve images and text
Answering questions about images and text
Making inferences or telling stories based on images and text
Summarizing large chunks of content that contain images and text
Generating essays and code based on images and text
Automating your dating life based on images and text
Building websites from almost nothing based on images and text
Showing you all the jobs it could replace based on images and text
Filing lawsuits based on images and text
Convincing a TaskRabbit worker to solve a CAPTCHA for it based on images and text
The paper also shows that GPT-4V can process arbitrarily interleaved multimodal inputs, meaning that it can handle any combination of images and text in any order. Moreover, the paper reveals that GPT-4V can understand visual markers drawn on input images, such as arrows, circles, or boxes, which can be used to refer to specific regions or objects in the image. This feature can enable new human-computer interaction methods, such as visual referring prompting.
The paper concludes with in-depth discussions on the emerging application scenarios and the future research directions for GPT-4V-based systems. The paper also acknowledges the challenges and limitations of GPT-4V, such as:
Generating inaccurate, outdated, or “hallucinated” information that requires verification
Having difficulties in understanding the context, logic, and nuances of human language
Raising ethical concerns and biases due to the quality and quantity of its training data
Being excessively verbose or easily steerable by the input
The paper suggests that these issues can be mitigated by using external knowledge sources, improving data quality and diversity, developing evaluation metrics and benchmarks, and applying human oversight and feedback.
I hope this summary helps you understand the paper better. If you want to read the full paper, you can find it here: The Dawn of LMMs: Preliminary Explorations with GPT-4V(ision).
Even if you don't read the paper in full, we strongly recommend following the link to explore the pages and pages of examples and use cases of the technology. We anticipate you'll be as surprised, and perhaps astonished, by the technology’s capabilities with images as you were the first time you used it for text. Here are a few examples of what it does well, and where things are in red, things it got wrong:
As parents we note, in the very least, that traditional math teaching will need to evolve. Students can now just take a photo of a problem and get an accurate answer. The examples above are just a taste. There is much, much more in the paper. Go see how GPT-4V can see.
That’s all we have for this edition of Confluence. We’ll leave you with something cool from our own trials with image creation in Bing chat. Given this prompt — “I would like you to create an image of someone working diligently on a modern piece of paper using a quill and inkwell. For this image I want you to use a creative styling that is entirely your own. Express yourself rather than trying to mimic some human style.” — Bing created four photographs. This one was particularly striking … note the technological symbols hanging from the end of the quill feathers …
AI Disclosure: We used generative AI in creating imagery for this post. We also used it selectively as a summarizer of content and as an editor and proofreader.
Bing chat’s description of general purpose technologies: General Purpose Technologies (GPTs) are technologies that can affect an entire economy (usually at a national or global level). GPTs have the potential to drastically alter societies through their impact on pre-existing economic and social structures. GPTs are usually pervasive, improve over time, and lead to complementary innovation.
Some examples of GPTs throughout history are the steam engine, electricity, information technology, the railroad, the automobile, and artificial intelligence. A GPT can be a product, a process, or an organizational system.
To be classified as a true GPT, a technology must meet four criteria:
It is a single, recognizable generic technology
It initially has much scope for improvement but comes to be widely used across the economy
It has many different uses
It creates many spillover effects
GPTs are often associated with productivity J-curves, which describe the phenomenon of initial decline followed by rapid increase in productivity after the adoption of a new GPT. This is due to the time required for development of new infrastructure, learning costs, and obsolescence of old technologies and skills.
GPTs also require the involvement of various functions and disciplines in their development and application, not just technical experts. This is because GPTs pose many ethical, social, and economic challenges that need to be addressed by diverse perspectives.
In economics this is called “Baumol Cost Disease.” Bing chat: Baumol Cost Disease is a phenomenon in economics that explains why some services become more expensive over time, even though their productivity does not increase. It is named after the economist William J. Baumol, who first described it in his study of the performing arts sector.
The basic idea is that some sectors of the economy, such as manufacturing or agriculture, experience high productivity growth due to technological improvements. This allows them to pay higher wages to their workers and lower their prices. However, other sectors, such as education, health care, or the arts, have low or no productivity growth, because they depend on human labor and skills that cannot be easily replaced by machines. These sectors still have to compete for workers with the high-productivity sectors, so they have to raise their wages as well. But since their productivity does not increase, they have to raise their prices to cover their higher costs.
This leads to a situation where the relative prices of services with low productivity growth increase over time compared to the prices of goods with high productivity growth. For example, a haircut or a concert ticket becomes more expensive relative to a computer or a car. This also means that the share of income spent on these services increases over time, which can have implications for public spending and social welfare.
Some possible solutions or mitigations for Baumol Cost Disease are increasing productivity in the low-productivity sectors through innovation or automation, subsidizing these sectors through public funding or donations, or reducing the demand for these services through education or regulation. However, these solutions may also have trade-offs or limitations, such as ethical, social, or cultural concerns. Therefore, Baumol Cost Disease remains a challenging issue for many economies and societies.