Confluence for 11.12.23
What you need to know about "drift." Indications of the impact of generative AI on white collar freelancers and working artists. The latest in disclosure and detection. What we've learned from GPTs.
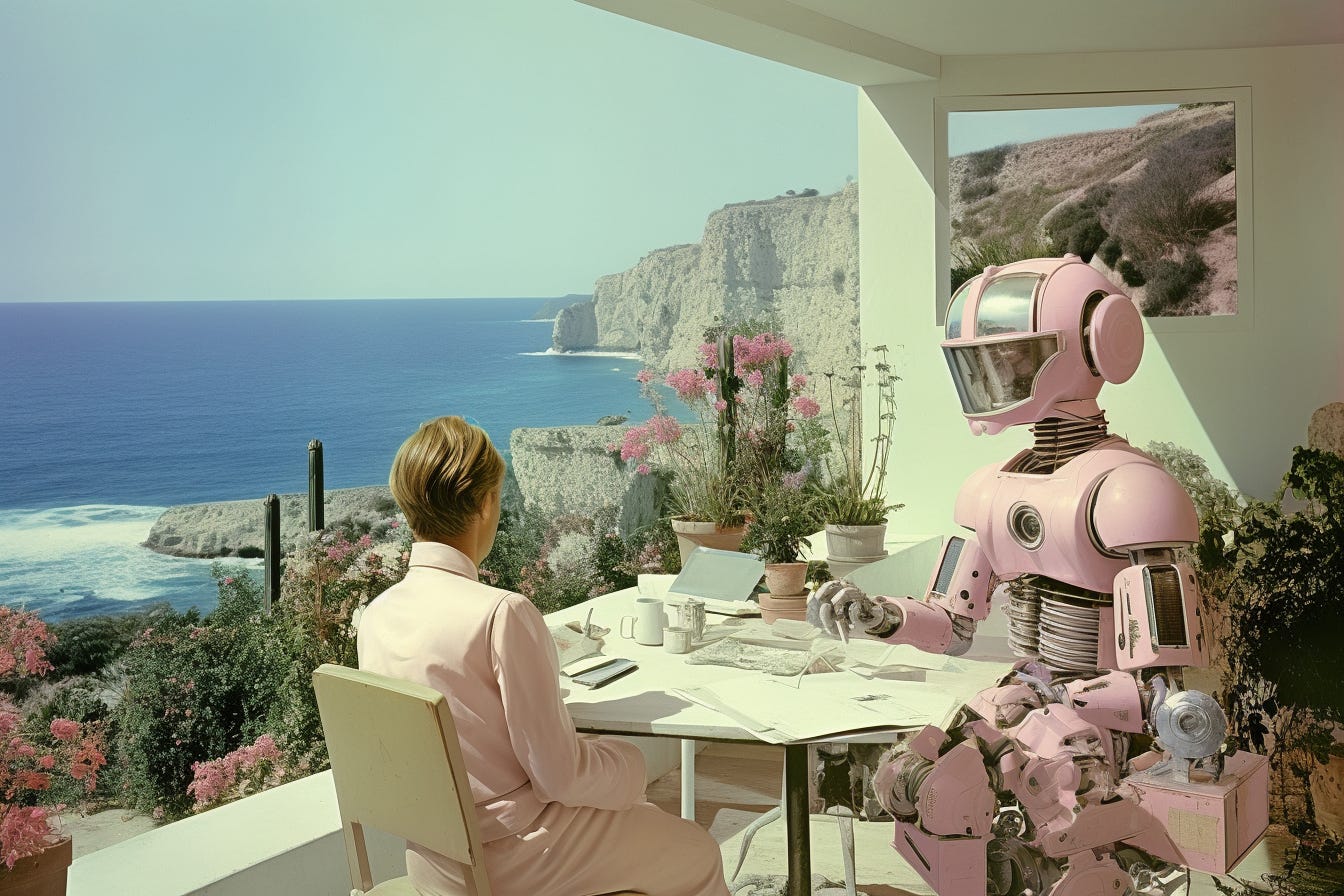
Welcome back to Confluence. We have two “above the bullets” items today. First is OpenAI’s Developer Day, where Sam Altman unveiled a number of groundbreaking new features. We shared our thoughts on those announcements in a separate post, and at the end of today’s issue we share how we’ve been experimenting with the new GPT features in the days since they became available. Second is a session we’re leading Nov. 30 and Dec. 1 on generative AI for communication professionals. It’s virtual, will span roughly two half-days (ending at 2:15 PM ET both days), and will offer a combination of big-picture perspective and hands-on training. You may download a one-page description of the session here, should you wish to learn more or attend. With those items out of the way, here’s what has our attention at the intersection of AI and communication:
What You Need to Know About “Drift”
Early Indications of the Impact of GenAI on Freelancers
A Study of the Impact of GenAI on Creativity in Working Artists
The Latest in Disclosure and Detection
What We’ve Learned from GPTs
What You Need to Know About “Drift”
This concept is critical to using generative AI in a helpful way, at least for now.
Lex Fridman’s most recent interview is with Elon Musk, whose X platform (nee Twitter) recently released the Grok AI application1. It’s interesting if you’re curious about Musk and how he thinks, but one part of the conversation in particular caught our attention. While talking about large language models and generative AI, Musk noted what he called, “drift,” and it’s a critical thing to understand if you’re going to be adept at using these technologies, at least for now.
The gist is this: without getting too technical, large language models like ChatGPT are prediction machines, laying out correlations between sets of letters, words, and concepts (which are vectors from a mathematical perspective) and using those to predict what letter, sets of letters, or words come next. In lots of ways, they are remarkably effective, and we (and probably you) are increasingly using them as part of our work. But every response is just a prediction. And all predictions involve some margin of error. In the first response in a chat, that error may be quite small — a great answer, or helpful response, with little or no confabulation. But as a chat or conversation with a tool like GPT-4, Claude 2, or Bing gets longer and longer, the error implicit in each response starts to accumulate. The vectors of the original response, which were quite accurate, have “drifted” over time, until they aren’t really pointing to where they were at the beginning.
We’ve certainly noticed this in our own work: while using GPT-4 to draft copy for an article, for example, we might start with a summary of a research paper, then ask GPT-4 to write longer-form piece based on our outline, and it will do a good job. We then give it a style guideline, and ask for another revision, and then ask it to re-do paragraph five … and before we know it, the copy we have is in fact worse than our first draft. The vectors drifted, the little errors accumulated, the thing got confused. As Musk noted in the interview, the model has predictive ability, but it lacks coherence, a larger gestalt about how the whole thing fits together. That’s very important to understand about these tools.
What does it mean for you day-to-day? Keep your chats short. Use them for a purpose, and then consider opening another chat for the next step of the process. We might use a chat to summarize an article, then a new chat to create a draft based on the outline, and then a third to revise to the style guide, and then a fourth for final proofreading. We’ll get less drift, and better output. At some point these models may solve the confabulation problem, but until then, having a sense of when they start to go astray is part of using them for maximum effect.
Early Indications of the Impact of GenAI on Freelancers
Some new data may be a harbinger of coming changes in the communication labor market.
When we first read the Harvard paper on the effects of generative AI use at Boston Consulting Group, one of the findings that most impressed us was the “upskilling” finding. This finding noted that the use of tools like ChatGPT made everyone participating in study better at their jobs, but that this increase was not proportional — the least-able employees benefited the most. Ethan Mollick writes more about that here, and you should read his take.
A first impression is that this is good news: large language models (LLMs) can make everyone better. But our very rapid second impression was related to the labor market: if you work in a role that has exposure to LLMs, and you’re, let’s say, “pretty good” at it, the introduction of LLMs in your professional space will make a lot of other people not as good as you also “pretty good,” and while the tools may also make you better, perhaps not with an equal (and differentiating) level of improvement. In essence, we presume these technologies have the ability to shift the distribution curve of talent in an exposed professional space, increasing the number of people who are roughly equally good at something. And when supply increases without an equal increase in demand, price drops. The bottom line: we think LLMs like ChatGPT have the ability to increase the labor and price competition for professionals, and free-lancers in particular, who compete in open labor markets. This is of particular importance in communication and its affiliated creative fields, where these labor markets are a large and important part of the professional supply chain.
Now we have two studies that seem to confirm this effect. The Short-Term Effects of Generative Artificial Intelligence on Employment: Evidence from an Online Labor Market, a working paper in the Munich Society for the Promotion of Economic Research, shows reductions in both the number of monthly jobs available to freelancers (2%) in occupations with significant LLM exposure, and a reduction in monthly earnings (5.2%), in an online labor market (Upwork) after the introduction of these tools. They were also 1.2% less likely to receive any employment in a given month and took 4.7% fewer jobs when employed. Further, and interestingly, the research found that freelancers who offered high-quality service, as measured by past performance and employment, were more affected by the introduction of AI — a finding worthy of deeper thinking. Second is a preliminary paper titled, Who is AI Replacing? The Impact of ChatGPT on Online Freelancing Platforms, which finds a 14% decrease following the introduction of ChatGPT in the number of job posts in jobs associated with writing, statistical analysis, electronic engineering, accounting research, web development, and other roles that have high exposure to generative AI when compared to jobs which require more manual tasks.
These percentages may seem small to some, and both studies focus on the short-term impact following the release of ChatGPT and other AI models and don’t capture long-term adaptations and evolutions in the labor market. That said, this is data worth noting given the size of the freelancer market in communication. We also think it may suggest broader implications for in-house communication roles where a large amount of the daily work has high exposure to generative AI.
The Impact of GenAI on Creativity in Working Artists
A new working paper explores some important questions with implications beyond the art world.
Generative AI tools like Midjourney, DALL-E, and Stable Diffusion have surged in popularity for their ability to create impressive and often striking imagery. As with other AI models and tools, their capabilities are rapidly improving, leading to increased adoption by amateur and professional creatives alike. A recent working paper by Boston University’s Eric Zhou and Dokyun Lee explores the impact these tools are having on artists’ creativity and productivity, analyzing activity and interaction on a large art sharing community. The study aimed to answer three questions:
How does the adoption of generative AI affect humans’ creative production?
Is generative AI enabling humans to produce more “creative” content?
When and for whom does the adoption of generative AI lead to more creative and valuable artifacts?
These are important, profound questions — the answers to which will have implications for fields beyond visual art. While we will not know the answers to these questions in a complete sense for a long time, the study’s findings on the current answers to these questions are informative for the creative aspects of fields beyond art (including corporate communications). A few of the findings jumped out to us as particularly relevant for our work:
Artists who used AI assistance produced 25% more artworks, and the perceived value of the artworks they produced (as measured by “favorites” on the platform) increased.
The average novelty in content and in style decreased over time among artists using AI.
Artists who can produce more novel ideas—regardless of how creative they were prior to using AI—received more favorable evaluations from their peers.
These are striking results, and it will be important to monitor these dynamics across creative fields as the capabilities of the tools and their adoption by professionals in a wide range of fields—artistic and otherwise—continues to increase.
What might this mean for corporate communications? We’ll find out, but here are some initial thoughts. First, generative AI tools, when used for the right things and in the right way, can increase both the volume and perceived quality of creative work. Second, we need to be wary of the risk of stylistic convergence and commoditization of content when using these tools (i.e., the tendency of ChatGPT or other tools to produce a lot of “pretty good” writing that sounds the same). Third, and perhaps most important, the ability to come up with novel good ideas—and to have the judgment and taste to effectively evaluate and curate the ideas of others—will be as important as ever.
The Latest in Detection and Disclosure
Don’t expect any definitive conclusions in this cat-and-mouse game anytime soon.
In previous Confluence entries and in our white papers from earlier this year, we’ve noted that as more and more content across a range of media is produced either in full or in part by AI, consumers of content will be increasingly skeptical as to its source. What has emerged is essentially a game of cat-and-mouse playing out on two levels: detection and disclosure.
The detection front is concerned with “catching” AI-generated content. Despite many companies making claims to the contrary, the latest evidence—including this study by Elkhetat, Elsaid, and Almeer published in The International Journal for Educational Integrity—suggests that AI detection tools are inconsistent at best, and that “the effectiveness of these tools may be limited in the fast-paced world of AI evolution.” At worst, as Eric Hauch argues, the tools can produce false positives that lead to reputational damage. Our current stance on the detection front aligns with the skeptics. With AI technology is evolving as quickly as it is, and with the interplay between humans and AI as complex and sophisticated as it is, you should—at the very least—be skeptical of anyone promising you a foolproof AI detection solution.
On the disclosure front, we continue to expect that norms will evolve over time and that no one can predict exactly what those norms will look like. That said, we do think it’s worth noting two important and potentially precedent-setting recent developments. First, in a long-expected move, Meta is requiring the disclosure of the use of generative AI in political or social issue ads, in an attempt to mitigate mis- and disinformation concerns ahead of the 2024 U.S. elections. Second, Google introduced a new feature called About This Image that “gives people an easy way to check the credibility and context of images they see online” by providing more information about an image’s history, how the image is used on other sites, and metadata (which includes fields that indicate generative AI use). The major players like Meta and Google will obviously play an outsized role in the norms that eventually cohere around disclosure, so it’s worth keeping an eye on these developments.
What We’ve Learned from GPTs
An early take on their immediate utility and potential long-term implications.
Since gaining access to GPTs (and the ability to create them) in ChatGPT earlier this week, we’ve spent time building our own and testing their capabilities. It’s still early days and there’s much more we’ll learn over the coming weeks, but the potential of GPTs is clearly enormous. Ethan Mollick shared a detailed explainer on GPTs earlier this week that is worth your time. That said, here are our early impressions and thoughts.
Prompt engineering is less important now that we have GPTs. In past editions of Confluence and in our white papers earlier this year, we’ve shared a number of thoughtfully designed prompts that professionals could copy and paste into their chats. GPTs remove a step in this process. Rather than maintaining your own running list of useful prompts, you can embed the same instructions within GPTs and share the link with whomever you want. Think of it as a way of pre-loading your conversations with any context you want to inform how AI responds to your requests. The bottom line is GPTs will make it much easier for less experienced users to tap into the full potential of existing (and future) tools.
GPTs will make it much easier to uphold consistent standards inside organizations. One concern we’ve heard among our clients is a potential “content explosion” as individuals across their organizations suddenly find it much easier to produce vast amounts of content. GPTs don’t eliminate that risk by any means, but they do make it possible to ensure that the content people work on meets certain standards. Rather than relying on individual judgment, teams can create GPTs designed to revise text or create graphics to align with a set of standards. We’ve already started to do this within our own firm, creating one GPT that can create logos and other graphics consistent with our branding and another that can copy edit based on our internal style guide and standards. To give you a sense of what this can look like, we’ve created a GPT designed to revise text based on The Elements of Style by Strunk and White for our readers to use.
While imperfect, GPTs are a sign of what’s to come. As powerful as GPTs are today, we are still finding limits to their abilities. But if there’s anything we’ve learned from the last year it’s that many or all of the limitations we experience today are only short-term. Given what we’ve learned and experienced working with GPTs this week, here’s how we are thinking about their future:
GPTs as tools. The most immediate application for GPTs is as a tool for communication professionals to create content and uphold standards. Tasks like copy-editing, developing first drafts of content, and creating visuals through GenAI tools will get easier and the barrier to entry will be lower. Communication teams will also be able to share their own GPTs with stakeholders that align to standards and expectations that all content shared within the organization meets.
GPTs as channels. Longer term, we see potential to use GPTs not just as tools for creating content, but as a way to communicate content with internal audiences. Because we can pre-load GPTs with specific context and information, it’s easy to imagine a world where organizations use GPTs to communicate directly with employees. Rather than emailing a 10 page FAQ guide or posting the same content to an intranet page, you could, in theory, upload the FAQ guide to a GPT then direct employees to share their questions via chat to get the answers they seek. While we’d encourage caution in the short-term given the potential for hallucinations, it doesn’t require a huge leap to imagine a future where GPTs become a critical communication channel within most organizations.
We’ll share more thoughts on GPTs in the coming weeks as we learn and experiment. If you have a GPT that you’ve built that you believe is useful, please share it in the comments.
Now we’ll leave you with something cool. We built a “No Bull Conversion Machine” GPT designed to eliminate corporate and technical jargon from your text. When you test it out, you’ll find that it has a bit of fun in the process.
AI Disclosure: We used generative AI in creating imagery for this post. We also used it selectively as a creator and summarizer of content and as an editor and proofreader.
Grok, especially in its “humorous mode,” is a foreshadowing of what’s to come: many, many large language models, integrated into a large number of platforms and applications — and increasingly with their own “personality,” style, or idiom.