Confluence for 4.28.24
A new custom GPT for you to use. Moderna scales GPT-4 use. Generative AI and automation bias. Accenture's report on generative AI.
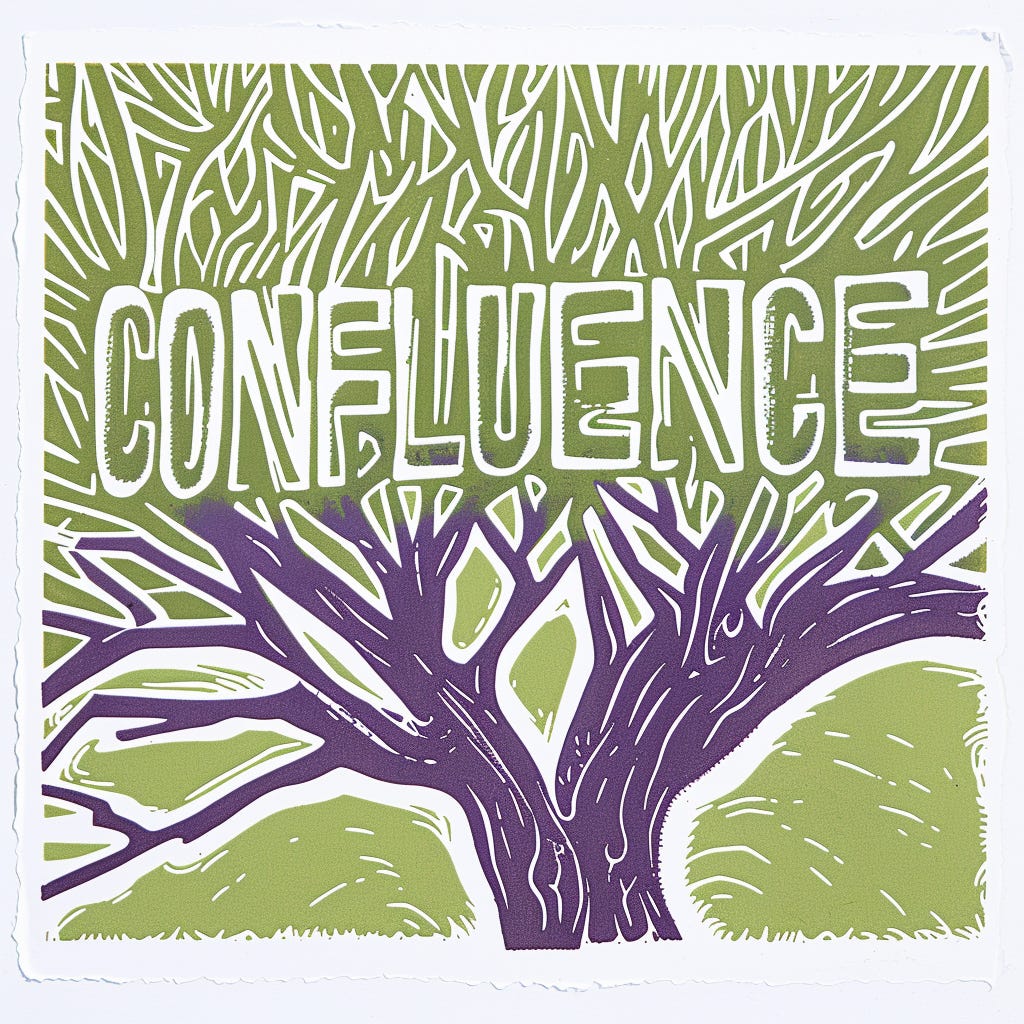
Welcome to Confluence. Here’s what has our attention at the intersection of generative AI and corporate communication this week:
A New Custom GPT for You to Use
Moderna Scales GPT-4 Use
Generative AI and Automation Bias
Accenture’s Report on Gen AI
A New Custom GPT for You to Use
“Project Definer” will help you define projects and risk mitigation strategies.
We have a relatively structured approach to defining a new engagement in our firm, which typically starts with a meeting among the project team to define a few important matters up-front. We learned long ago that creating clarity early on goes a long way in saving time and dealing with ambiguity later. To help us do this more easily, and to help folks in our firm who may leading a project as a sole team member, we recently created a custom GPT that automates the process for us. It helps the user:
Define the purpose and vision of the project,
Identify key principles or rules of engagement that the project team should follow, and
Identify and define successful outcomes for the key stakeholders of the effort.
In addition, the GPT also engages the user (or team, if a team is using it in a project definition meeting) in a “Crystal Ball” exercise, a planning approach the military often uses that we picked up long ago. In essence, it involves imagining you have a crystal ball that can predict the future. You ask the crystal ball if the project succeeds or fails, and it tells you that it failed. You then offer a reason for failure: “Was it because our team was disconnected?” The ball replies, “No,” which forces you to offer another possible reason for failure. In essence, it’s a way of conducting a pre-mortem on a project to imagine potential risks so you can plan ahead with mitigation strategies. The custom GPT plays the role of the crystal ball, helping you identify reasons for failure and mitigation strategies for each — and if you ask, it will even offer mitigation strategies in addition to those you might think of.
At the end of the process, the GPT produces a project definition report that lists all the input under helpful headings. It even gives the user the choice to download the report as a Word document.
This is a very helpful little GPT, and it’s an excellent example of how custom instruction sets can automate all sorts of routines that individuals and teams may otherwise complete in more manual ways. And the crystal ball segment is a great example of how a large language model, given a little structure, can play the role of a helpful collaborator in brainstorming ideas (or in this case, risks and ways to mitigate them).
While we designed the “Project Definer” GPT for use in our firm, we’ve set the permissions so that it’s available to anyone with a GPT Plus or GPT Team account. You may use it here, and we hope you find it helpful.
Moderna Scales GPT-4 Use
The pharma company is all-in on generative AI via a partnership with OpenAI.
We are fortunate that our firm, after over 35 years in business, has an extensive client list that includes many of the world’s largest organizations, but that also spans a wide spectrum from small privately held firms to large public-sector multilaterals. And yet, for all that diversity, most of our clients have at this point only dipped their toes in the water of generative AI use. This is one reason we were surprised to learn this week of Moderna’s all-in commitment to generative AI, and OpenAI’s ChatGPT in particular, via a continuing partnership between the firms.1 This video gives an overview:
The relationship started in early 2023 and now involves the widespread use of ChatGPT Enterprise across the company. Moderna has over 750 custom GPTs in use across the company, 100% adoption of ChatGPT and other large language models in the legal department, and the average user has 120 ChatGPT conversations per week (or an average of 24 per day). You can read OpenAI’s case study on the work here, and the Wall Street Journal has a story here. From that story, it seems Modern aims to automate nearly every business process at the company, in part through ChatGPT, and to immediately make ChatGPT Enterprise available to (as near as we can tell) all of its over 3,000 employees.
With Moderna being an early adopter of this technology at scale, we’re certain there will be much to learn from their experience. Reading the case study, it seems they — rightly — invested significant energy in the change management side of this effort. But we’re also left with this observation: if they can figure out how to do it, with all the regulation and risk that comes with drug development, we presume just about anyone can. So, in this, Moderna may be a harbinger of things to come.
Generative AI and Automation Bias
We continue to learn more about using these tools every day — and this includes learning from our own mistakes.
In his book Co-Intelligence, Ethan Mollick writes the following about how anchoring bias can play out in our use of generative AI tools:
When we use AI to generate our first drafts, we tend to anchor on the first idea that the machine produces, which influences our future work. Even if we rewrite the drafts completely, they will still be tainted by the AI’s influence. We will not be able to explore different perspectives and alternatives, which could lead to better solutions and insights.
We immediately recognized the accuracy and power of that insight, and it led us to wonder: what other cognitive biases might come into play when using these tools? We asked Claude 3 Opus, which generated the following list:
Automation bias — The tendency to favor suggestions from automated systems and to ignore contradictory information made without automation, even if it is correct. People may put too much trust in AI outputs.
Confirmation bias — The tendency to search for, interpret, favor, and recall information in a way that confirms or supports one's prior beliefs or values. If an AI output aligns with what someone already believes, they may favor it regardless of its actual quality or accuracy.
Focalism / anchoring effect — The tendency to rely too heavily on the first piece of information offered (the "anchor") when making decisions. As Mollick points out, people tend to fixate on and iterate from the initial AI-generated text or image rather than asking for many diverse outputs.
Availability bias — The tendency to overestimate the likelihood of events with greater "availability" in memory. If AI easily generates vivid examples of something, it may lead people to think it is more prevalent than it really is.
Mere exposure effect — The tendency to develop a preference for things merely because of familiarity with them. The more someone interacts with an AI, the more they may grow to like and trust it, even if that trust is not fully warranted.
Authority bias — The tendency to attribute greater accuracy to the opinion of an authority figure and be more influenced by that opinion. People may view AI as an authoritative source and put undue stock in what it generates.
Interestingly enough, automation bias was both at the top of Claude’s list2 and at top of mind for us. Eagle-eyed readers may have noticed a few typos in last week’s edition of Confluence. We used Claude to proof (following the best available human standard), in addition to having one of our team give it a final read. Both we and Claude missed the typos, and we trusted Claude more than we should have — an example of the automation bias in action.
While we were certainly kicking ourselves a bit when we realized our errors, it’s a good reminder that, despite their impressive capabilities, large language models are not infallible — and neither are we when we rely on them too heavily. Maintaining human oversight and critical thinking is crucial to harnessing their benefits while avoiding the pitfalls of falling asleep at the wheel.
Accenture’s Report on Generative AI
Accenture’s report reveals disconnect between c-suite and employees on generative AI.
While Accenture’s report on generative AI reads largely as we’d expect given what we’ve seen from similar firms on the topic, there is data within it that caught our attention. Accenture highlights a significant gap between how C-suite executives and employees perceive the effects of generative AI on the workforce. The findings suggest that leaders may be underestimating employees’ concerns and insecurities as organizations increasingly integrate generative AI into how they operate.
According to Accenture’s research, 58% of workers believe that generative AI is increasing their job insecurity, and 60% are concerned that it may lead to increased stress and burnout. In contrast, only 29% of C-suite executives believe that job displacement is a concern for their employees, and just 37% think that generative AI could contribute to employee stress and burnout.
This disconnect between leadership and employees presents an opportunity for communication professionals to help shape the narrative around generative AI within their organizations. To do this effectively, communicators should understand both the fundamentals of the technology, employee beliefs about it, and what it potentially means for them. If Moderna’s approach to integrating generative AI across the organization at scale becomes a model for others, communication professionals need to be prepared to help employees make sense of the significant changes that may reshape their work and the organization in a way that speaks to their questions and concerns.
We’ll leave you with something cool: Reid Hoffman engaging in a conversation with an AI-generated version of himself.
AI Disclosure: We used generative AI in creating imagery for this post. We also used it selectively as a creator and summarizer of content and as an editor and proofreader.
Disclosure: Moderna is not an active client of our firm.
So as not to be too limited by the anchoring bias and constrained to the first list of biases, we ran the prompt again. Claude generated a similar but slightly different list, though on both lists, automation bias was listed first. Here’s the second list:
Automation bias: People may trust the outputs of an AI system more than they should, assuming that the AI is always correct or more accurate than human judgment.
Anthropomorphism bias: Users may attribute human-like characteristics, emotions, or intentions to the AI chatbot, leading to unrealistic expectations or misinterpretations of the AI's responses.
Confirmation bias: People may seek information from the AI that confirms their preexisting beliefs or hypotheses, while disregarding information that contradicts them.
Availability bias: Users might rely on the information that is most readily available from the AI, rather than considering a broader range of sources or perspectives.
Framing effect: The way questions are phrased or the context in which they are asked can influence the AI's responses, which in turn may affect the user's perception and decision-making.
Illusion of explanatory depth: Users may believe they understand the AI's reasoning or the topic under discussion better than they actually do, based on the AI's responses.
In-group bias: If the AI chatbot is associated with a particular brand, organization, or ideology, users may be more likely to trust and accept its responses due to their affiliation with that group.
Recency bias: People may give more weight to the most recent information provided by the AI, even if it is less relevant or accurate than earlier information.